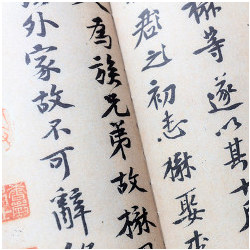
In the past decades, one line has run through the entire research spectrum of natural language processing (NLP)—knowledge. With various kinds of knowledge, such as linguistic knowledge, world knowledge, and commonsense knowledge, machines can understand complex semantics at different levels. In this article, we introduce a framework named “knowledgeable machine learning” to revisit existing efforts to incorporate knowledge in NLP, especially the recent breakthroughs in the Chinese NLP community.
Since knowledge is closely related to human languages, the ability to capture and utilize knowledge is crucial to make machines understand languages. As shown in the accompanying figure, the symbolic knowledge formalized by human beings was widely used by NLP researchers before 1990, such as applying grammar rules for linguistic theories3 and building knowledge bases for expert systems.1 After 1990, statistical learning and deep learning methods have been widely explored in NLP, where knowledge is automatically captured from data and implicitly stored in model parameters. The success of the recent pretrained language models (PLMs)4,13 on a series of NLP tasks proves the effectiveness of this implicit knowledge in models. Making full use of knowledge, including both human-friendly symbolic knowledge and machine-friendly model knowledge, is essential for a better understanding of languages, which has gradually become the consensus of NLP researchers.
Figure. A historical glimpse of the NLP research spectrum and the whole framework of knowledgeable machine learning.
The spectrum depicted in the figure shows how knowledge was used for machine language understanding in different historical periods. The framework shows how to inject knowledge into different parts of machine learning.
Knowledgeable ML for NLP
To clearly show how to utilize knowledge for NLP tasks, we introduce knowledgeable machine learning. Machine learning consists of four components: input, model, objective, and parameter. As shown in the figure, knowledgeable machine learning aims at covering the methods that apply knowledge to enhance these four machine learning components. According to which component is enhanced by knowledge, we can divide existing methods utilizing knowledge for NLP tasks into four categories:
Knowledge augmentation enhances the input of models with knowledge. There are two mainstream approaches for knowledge augmentation: one is to directly add knowledge into the input, and the other is to design special modules to fuse the original input and related knowledgeable input embeddings. So far, knowledge augmentation has achieved promising results on various tasks, such as information retrieval,11,18 question answering,10,15 and reading comprehension.5,12
Knowledge support aims to bolster the processing procedure of models with knowledge. On one hand, knowledgeable layers can be used at the bottom for preprocessing input features, and features can thus become more informative, for example, using knowledge memory modules6 to inject informative memorized features. On the other hand, knowledge can serve as an expert at top layers for post-processing to calculate more accurate and effective outputs, such as improving language generation with knowledge bases.7
Knowledge regularization aims to enhance objective functions with knowledge. One is to build extra objectives and regularization functions. For example, distantly supervised learning utilizes knowledge to heuristically annotate corpora as new objectives and is widely used for a series of NLP tasks such as relation extraction,8 entity typing,17 and word disambiguation.9 The other approach is to use knowledge to build extra predictive targets, such as ERNIE,20 CoLAKE,14 and KEPLER,16 which take knowledge bases to build extra pre-training objectives for language modeling.
Knowledge transfer aims to obtain a knowledgeable hypothesis space and make it easier to achieve effective models. Both transfer learning and self-supervised learning focus on transferring knowledge from labeled and unlabeled data respectively. As a typical paradigm of transferring model knowledge, fine-tuning PLMs has shown promising results on almost all NLP tasks. Some Chinese PLMs like CPM21 and PanGu-alpha19 have recently been proposed and have shown awesome performance on Chinese NLP tasks. CKB2 has further been proposed to build a universal continuous knowledge base to store and transfer model knowledge from various neural networks trained for different tasks.
Since knowledge is closely related to human languages, the ability to capture and utilize knowledge is crucial to make machines understand languages.
Besides the studies mentioned here, many researchers in the Chinese NLP community are committed to using knowledge to enhance NLP models. We believe all these efforts will advance the development of NLP toward better language understanding.
Conclusion
In this article, we introduced a knowledgeable machine learning framework to show existing efforts of utilizing knowledge for language understanding, especially some typical works in the Chinese NLP community. We hope this framework can inspire more efforts to use knowledge for better language understanding.
Join the Discussion (0)
Become a Member or Sign In to Post a Comment