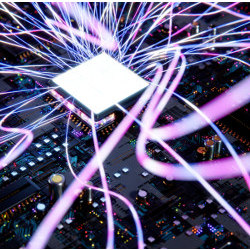
The success of machine learning for a wide range of applications has come with serious costs. The largest deep neural networks can have hundreds of billions of parameters that need to be tuned to mammoth datasets. This computationally intensive training process can cost millions of dollars, as well as large amounts of energy and associated carbon. Inference, the subsequent application of a trained model to new data, is less demanding for each use, but for widely used applications, the cumulative energy use can be even greater.
“Typically there will be more energy spent on inference than there is on training,” said David Patterson, Professor Emeritus at the University of California, Berkeley, and a Distinguished Engineer at Google, who in 2017 shared ACM’s A.M. Turing Award. Patterson and his colleagues recently posted a comprehensive analysis of carbon emissions from some large deep-learning applications, finding that energy invested to refine training can be more than compensated by reduced inference costs for improved models.
The paper also notes that users can reduce carbon dioxide (CO2) emissions beyond those from energy savings by choosing less carbon-intensive electricity sources for their calculations. “If you pick a solar-powered energy grid versus an Australian coal-based one, you can reduce your emissions by 80-fold,” said Alexandra “Sasha” Luccioni, a postdoc at the AI-focused Mila Institute in Quebec, Canada, who previously published a tool to help users estimate their carbon footprints. In many cases, Luccioni suspects this reduction will more than compensate for the energy needed to transfer the data to a remote location, but those trade-offs need to be quantified.
Specialized Accelerators
The amount of energy devoted to computing is now significant on a global scale. Historically, increasing power requirements were largely offset by more efficient technologies in accord with Moore’s Law, and manufacturers continue to introduce innovative new generations of technology. Since the early 2000s, however, critical device parameters such as operating voltage could no longer follow the classic scaling strategy, and the rate of power improvement has slowed, said Jonathan Koomey, president of Koomey Analytics, who has consulted with manufacturers to validate their energy goals.
Nonetheless, “There are ways around it, at least for a time,” he said. “These ways around it sometimes involve better software, sometimes involve optimization of hardware and software, and sometimes it involves special-purpose computing devices to do particular tasks much more quickly than a general-purpose computer could do.”
Web services providers, as well as companies that do their own processing, increasingly employ hardware accelerators that are specialized for deep learning, and startup companies have moved to exploit this opportunity. These accelerators are aimed primarily at speeding calculations, but they also significantly reduce energy consumption by reducing the number of unnecessary operations and data transfers,
The neural networks underlying deep learning comprise multiple layers of units reminiscent of brain cells, in that each connects to many similar units. The activity of each “neuron” is calculated from the sum of the activity of many others, multiplied by an adjustable “weight.” Training tunes these weights so the output approaches the desired one for each input, and may also explore alternative “models,” meaning the interconnections and responses of neurons.
The required calculations can be done using a general purpose central-processing unit (CPU), which is convenient for small training tasks. As the tasks get larger and more mature, however, there are strong motivations—both speed and energy efficiency—to take advantage of the predictability and parallelism of the calculation by adding specialized hardware.
Many users utilize graphics-processing units (GPUs) for acceleration, especially during training. Although these devices were developed for image rendering and display tasks, their highly parallel structure, optimized for multiply-accumulate operations, make them well-suited for neural networks. That market is dominated by NVIDIA and Advanced Micro Devices, which now market devices expressly for artificial intelligence applications. The most advanced GPUs combine powerful processor chips with memory in a single advanced package that supports high-bandwidth communications.
Some users, notably Microsoft, continue to champion flexible field-programmable gate arrays (FPGAs) for deep learning. In 2015, however, Google, motivated by dire predictions of the energy servers could need for voice processing inference, introduced the first version of its Tensor Processing Unit (TPU).
These custom chips were created specifically for deep-learning inference using “ASIC” design tools. However, said Patterson, “The problem is that the abbreviation Application Specific Integrated Circuit makes it sound like you’re building hardware that can only do one particular model.” Actually, TPUs and other accelerators, including GPUs, can assist a variety of models.
Companies are pursuing various approaches. Cerebras, for example, has gotten a lot of attention for using an entire silicon wafer for a chip, including dozens of processing units. Nonetheless, the rapid advances and growth of models poses a challenge for dedicated hardware that embodies specific assumptions about computations, memory distribution, and communications. Recently, for example, researchers have had great success with “transformers” such as OpenAI’s massive language model GPT-3, which has more than 100 billion parameters.
Big Opportunities
Despite these challenges and the dominance of corporate giants, smaller companies have sensed opportunities for hardware innovation. “I don’t think in my career I’ve seen so many hardware start-ups sprout up over such a short period of time,” said David Brooks, Haley Family Professor of Computer Science at Harvard University. “That’s a good thing, but there will be shaking out in the process,” demonstrated by the companies that already have folded, or shifted their strategies.
Device designers use a variety of techniques to skip unnecessary operations. A key strategy exploits the “sparsity” of many models. “There end up being a lot of zeroes in the weight matrixes,” said Brooks. “If you can find ways to find all the zeroes, and then avoid having to send it through the whole data path, you can save quite a lot” of energy by skipping them. Other forms of sparsity also can be exploited, he said. For example, “There are a lot of very small values that may as well be a zero.”
“The biggest thing that I’ve been encountering is they’re decreasing their precision at an instruction-set level,” said Andrew Lohn, an analyst at the Center for Security and Emerging Technology at Georgetown University. “They’re able to have faster, more efficient operations, because deep learning applications don’t need all of that precision,” especially for inference.
The rapid advances and growth of models poses a challenge for dedicated hardware that embodies specific assumptions about computations, memory distribution, and communications.
Patterson also highlights the importance of memory design, including locating SRAM where it is needed on the chip, as well as high-bandwidth connections to off-chip DRAM. Although arithmetic units are important, he said, “Where the energy and the time is going is memory access.”
The innovators often focus on hardware, and “Many of them did not invest in the software stack,” Patterson said. For the most part, companies have not reported results for benchmarks like the MLPerf suite, which Patterson said he worried “is a really bad sign.”
Optimizing how the hardware works together with a particular model could push users to a more comprehensive design process, Brooks said. “Codesign in some sense is all about breaking abstractions and trying to design things that are across multiple layers of the stack.” Ironically, he said, “Machine learning is perhaps a good way of breaking some of those abstractions,” relieving some of the problems it causes.
Hyperscale Computations
The full energy and carbon impact of AI includes not only accelerator chips, but off-chip and long-distance data transfer, as well as the large energy overhead of the facility infrastructure, such as cooling and power supplies. “Google has definitely been on the cutting edge of improving things in terms of efficiency,” Koomey said. The search engine giant reports excess energy of only about 10% of the computational energy in their hyperscale datacenters, which he said is substantially lower than some other facilities, especially underutilized corporate servers.
Lack of transparency about energy use, sometimes for competitive reasons, remains a problem. Koomey has argued that limited information has contributed to some misleadingly pessimistic estimates of AI energy use.
Indeed, although hosting many calculations lets providers improve utilization, “When you’re running on a large cluster, it’s hard to isolate the energy consumption of a given process or a given user,” said Mila’s Luccioni. Publications often omit other important details, and she and her colleagues developed their assessment tool after they found it impossible to glean them from papers. She also worries about the energy and carbon costs of making the devices in the first place. “We have no figures at all what kind of CO2 is emitted creating an NVIDIA GPU.”
Luciani expressed hope that standardized disclosure of energy and carbon impacts would become a common requirement for publications and conferences, like the posting of code and data to promote reproducibility.
Sze, V., Chen, Y., Yang, T., and Emer, J.S.
How to Evaluate Deep Neural Network Processors, ISSCC 2020 Tutorial, https://bit.ly/2ZAHMhg
Patterson, D., Gonzalez, J., Le, Q, Liang, C., Munguia, L., Rothchild, D., Texier, M., and Dean, J.
Carbon Emissions and Large Neural Network Training, [currently posted as a preprint on ArXiv, but under review at CACM, so it may be printed before this story] (2021), https://arxiv.org/abs/2104.10350
Khan, S.M. and Mann, A.
AI Chips: What They Are and Why They Matter, Center for Security and Emerging Technology (2020), https://bit.ly/3beaC9u
Koomey J. and Masanet, E.
Does not compute: Avoiding pitfalls assessing the Internet’s energy and carbon impacts, Joule 5, 1 (2021), https://bit.ly/3bbeiJh
Join the Discussion (0)
Become a Member or Sign In to Post a Comment